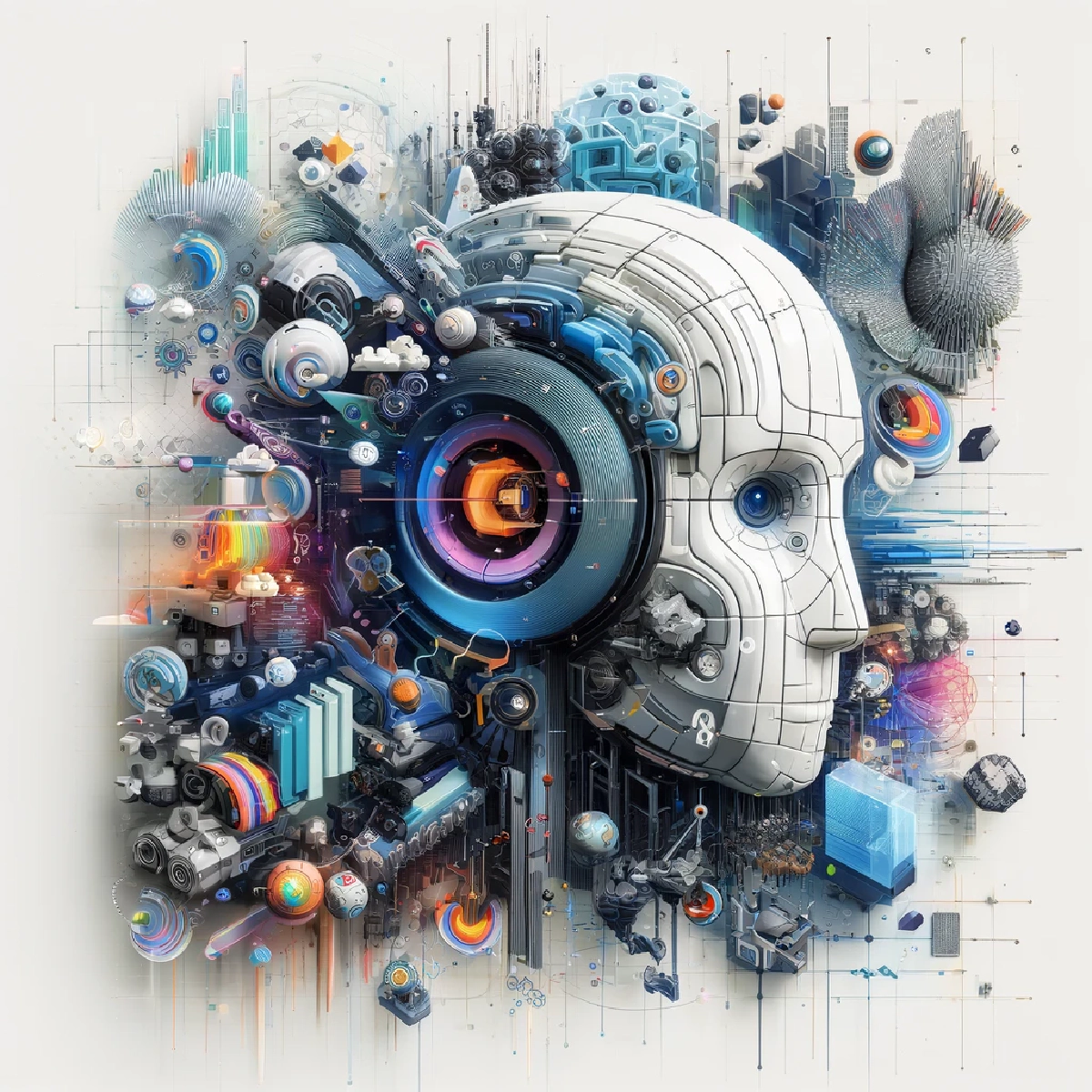
Deep Reinforcement Learning: Applications & Challenges
10 Min read
Deep Reinforcement Learning (DRL) stands at the forefront of AI, bridging the gap between the potential of artificial …
Artificial intelligence (AI) can significantly improve healthcare industry in the way of enhancing diagnostic accuracy, optimizing treatment plans, and improving operational efficiencies. AI technologies, such as machine learning and deep learning demonstrated capability to analyze vast amounts of data much faster than regular humans, enabling quicker and more precise diagnostics. In this article, we will explore AI impact on the medial sector
Artificial intelligence boosts operational efficiency for healthcare industry. This is achieved through algorithms optimized for pattern recognition and predictive analytics, where AI can manage resource allocation, patient scheduling, and inventory controls, reducing waste and improving service delivery. AI systems can predict peak times for patient visits, helping hospitals staff appropriately and manage patient flow more effectively. This level of automation also extends to back-office operations, automating billing and claims processing, which enhances financial operations.
In terms of UI/UX ai is really a good option to provide personalized patient engagement through interactive tools like chatbots and virtual assistants. These AI-driven platforms offer immediate responses to patient inquiries, schedule appointments, and help manage chronic conditions, improving overall satisfaction. Enhanced user experience leads to better adherence to treatment plans and increased patient trust in healthcare providers. At the end generative AI can be used to prepare content and educate patients on their health conditions, thereby fostering a proactive approach to health management.
AI plays a crucial role in improving diagnostic accuracy and speed, particularly in image-based diagnostics such as radiology and pathology. Machine learning models analyze medical imaging faster and often more accurately than human counterparts, leading to quicker, more efficient patient care. These AI systems also reduce the rate of false negatives and false positives, important for conditions such as cancer where early detection is key. They support pathologists by highlighting areas of interest on slides, potentially identifying disease features missed by the human eye.
graph LR data[Data Collection] --> AI{AI Processing} AI --> patterns[Pattern Recognition] AI --> predictions[Predictive Analytics] patterns --> management[Resource Management] predictions --> scheduling[Patient Scheduling] management --> optimization[Operational Optimization] scheduling --> efficiency[Increased Efficiency]
Wearable AI-driven devices monitor vital signs and detect deviations, alerting users and healthcare providers to potential health issues before they escalate. Continuous monitoring supports preventive healthcare, significantly reduces the risk of emergency medical events and in addition, these devices can collect and analyze data over time, providing insights into patient health trends and provinding early signals about health trends. This technology is pivotal for chronic disease management, helping to adjust treatments based on the collected data.
AI’s ability to aggregate and analyze data from diverse sources, including electronic health records (EHRs), genomics, and wearable devices, allows comprehensive management. This integration helps healthcare providers deliver personalized treatment plans and improves outcomes. AI’s data integration capabilities also extend to predictive health risks assessments, identifying at-risk patients based on various biomarkers and historical data. Moreover, AI systems can suggest optimal treatment approaches based on similar patient profiles and clinical outcomes.
AI systems enhance early detection and diagnosis of diseases, particularly in oncology, by analyzing patterns in data that may elude human observers. These AI-driven insights facilitate early intervention, which can be crucial for patient prognosis. Such tools can screen through vast datasets quickly, identifying abnormalities in earlier stages than currently possible with traditional methods. They also help in cross-referencing patient histories and current tests, ensuring a comprehensive analysis.
Machine learning systems is a major contributor to precision medicine by utilizing algorithms that predict which treatment protocols are most effective based on individual patient data, including genetic information. This tailored approach not only enhances efficacy but also minimizes side effects. AI’s role in precision medicine extends to the development of new drugs, where it can predict molecule interactions faster than traditional experimental methods. It also helps in simulating clinical trials, speeding up the drug approval process.
AI assists in developing and optimizing treatment plans using predictive analytics to assess potential outcomes. This supports actual workers of the industry and professional medics in making informed decisions that align with best practices and patient-specific factors. Continuously updates and refines of treatment plans based on ongoing patient data, ensuring that care remains dynamic and responsive to individual needs - is also a nice capability AI can share. These systems integrate various data types, from clinical studies to real-time monitoring data, to provide comprehensive support in decision-making.
Robots are modern trend. They are reality and not fantasies. Robotic systems improve the precision of surgeries, reduce the risk of infection, and shorten recovery times. These robots can perform complex procedures with a level of consistency and accuracy that enhances patient safety and treatment outcomes. Additionally, AI in robotics facilitates minimally invasive techniques, which are less traumatic for patients and lead to faster recovery. The use of AI also extends to post-operative care, where it monitors patients and adjusts treatments as needed, based on the recovery progress.
AI enhances post-operative care and chronic disease management by providing continuous remote monitoring and real-time data analysis, which helps adjust treatment plans as needed and alert healthcare providers to changes in patient conditions. AI systems integrate data from multiple sources, providing a holistic view of a patient’s health and enabling more precise adjustments to care plans. These systems also support healthcare providers by offering predictive insights into patient deterioration, allowing preemptive interventions.
Apps leveraging AI technology offer diagnostic support, treatment options, and management for diseases like diabetes and depression, enhancing accessibility and effectiveness of medical care. These apps are not only user-friendly but also integrate seamlessly with existing healthcare systems, providing a bridge between patients and healthcare services
The integration of AI into healthcare faces challenges including data privacy, ethical concerns, and the need for extensive training data to develop accurate AI models. Overcoming these hurdles is essential for maximizing the benefits of AI in healthcare. Additionally, there are technical challenges in terms of integrating AI with legacy systems in hospitals, and there is a persistent need for clinical validation of AI tools to ensure they meet regulatory standards.
The future of AI in healthcare looks promising with ongoing advancements leading to more integrated, predictive, and personalized care. The evolution of AI technologies is expected to further transform the landscape of healthcare delivery and management. Continued research and development are expected to address current limitations, leading to broader adoption across all areas of healthcare.
AI increases responsibility by enhancing diagnostic accuracy, treatment efficacy, and overall healthcare management, ensuring higher standards of care. It also brings a new level of transparency and data-driven decision-making to healthcare, which is crucial for patient trust and system reliability.
AI has achieved high levels of accuracy in various applications, particularly in diagnostic imaging, often surpassing traditional methods. The precision of AI systems continues to improve as they learn from more data, making them increasingly reliable partners in healthcare settings.
AI represents a transformative development in healthcare, promising to enhance all aspects of patient care and system efficiency. It offers significant advantages in terms of scalability, speed, and cost-effectiveness, which are critical for addressing the growing demands of global health systems.
AI will not replace humans in healthcare but will augment the capabilities of healthcare professionals, allowing them to deliver more effective and efficient care. AI is best seen as a tool that enhances human skills and allows healthcare workers to focus more on patient care rather than administrative tasks.
AI solves numerous problems, including inefficiencies in healthcare delivery, diagnostic errors, and the lack of personalized treatment, significantly improving the quality and accessibility of care. It also addresses challenges related to data overload by providing tools that synthesize and interpret vast amounts of health data, enabling better clinical decision-making.
10 Min read
Deep Reinforcement Learning (DRL) stands at the forefront of AI, bridging the gap between the potential of artificial …
10 Min read
Introduction to Automated Weapon Systems Automated weapon systems, also known as autonomous weapons or lethal autonomous …
Looking for a solid engineering expertise who can make your product live? We are ready to help you!
Get in Touch